Traditional cache solutions treat each entry as an immutable blob of data, which poses problems for the append-heavy ingestion workloads that are common in Pravega. Each Event appended to a Stream would either require its own cache entry or need an expensive read-modify-write operation to be included in the Cache. To enable high-performance ingestion of […]
The ability to pipeline Events to the Segment Store is a key technique that the Pravega Client uses to achieve high throughput, even when dealing with small writes. A Writer appends an Event to its corresponding Segment as soon as it is received, without waiting for previous ones to be acknowledged. To guarantee ordering and […]
Streaming applications typically need to process the events as soon as they arrive. For example, being able to quickly react to events in applications such as fraud detection, manufacturing error detection can result in massive savings. However, due to the limitation of storage systems not being able to handle large numbers of small writes, producers […]
The Pravega Segment Store Service is a subsystem that lies at the heart of the entire Pravega deployment. It is the main access point for managing Stream Segments, providing the ability to modify and read their contents. The Pravega Client communicates with the Pravega Stream Controller to identify which Segments need to be used (for […]
Several of the difficulties with tailing a data stream boil down to the dynamics of the source and of the stream processor. For example, if the source increases its production rate in an unplanned manner, then the ingestion system must be able to accommodate such a change. The same happens in the case a processor […]
Introduction Reading and writing is the most basic functionality that Pravega offers. Applications ingest data by writing to one or more Pravega streams and consume data by reading data from one or more streams. To implement applications correctly with Pravega, however, it is crucial that the developer is aware of some additional functionality that complements […]
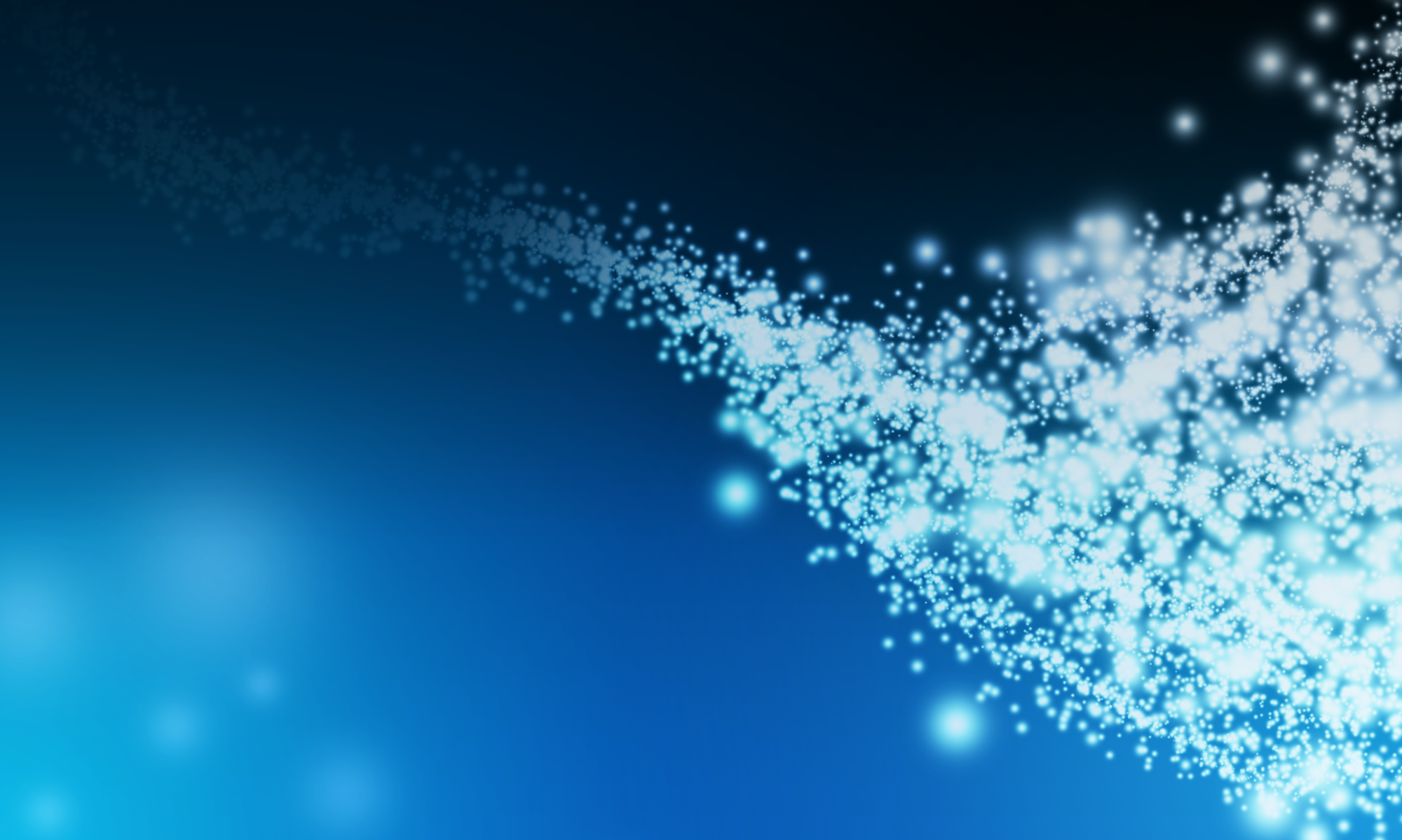
Driven by the desire to shrink to zero the time it takes to turn massive volumes of raw data into useful information and action, streaming is deceptively simple: just process and act on data as it arrives, quickly, and in a continuous and infinite fashion. For use cases from Industrial IoT to Connected Cars to […]